ChatGPT is not just lossy compression
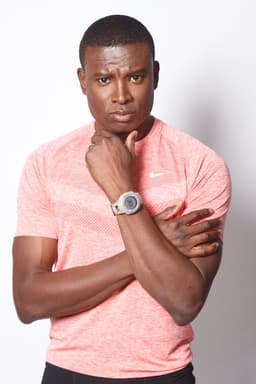
Emily Thompson
Published on December 21, 2023
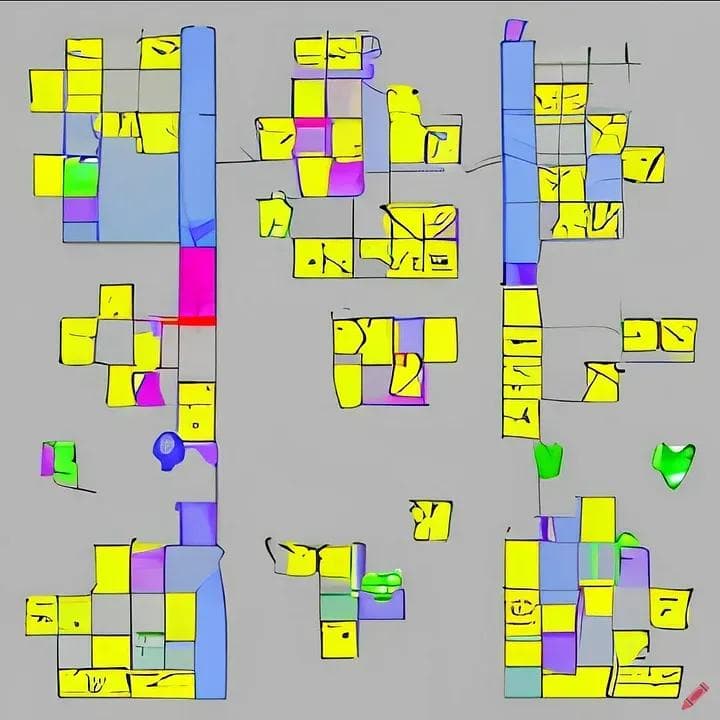
Recently, an interesting and stimulating criticism of large language models like OpenAI’s ChatGPT and addressed to the general public has been published in the New Yorker, comparing them to lossy compression for images. In this article, the author, Ted Chiang (henceforth TC), argues that language models are not capable of producing original content, and that they are merely a lossy compression of all the text on the web, the lossy aspect being dangerous because difficult to detect like pointed out by David Kriesel during his investigation of the infamous Xerox bug [1] [2]. However, this view overlooks many of the capabilities of language models and oversimplifies their purpose and limitations.
In an engineering spirit, this post will address the flaws in this specific comparison of LLMs to lossy compression and provide a more nuanced and down-to-earth view of the capabilities and limitations of language models. We will argue that language models are not simply a lossy compression of text data, let alone image data, but are instead interactive systems designed for a wide range of tasks, including natural language understanding and generation, question-answering, and more.
Furthermore, we will demonstrate that the fallibility of language models, like that of plausible hallucination, is not a weakness but rather a reflection of the complexity and variability of natural language. And while there are limitations to these models, there are also countermeasures in place to address these, such as fine-tuning on specific tasks or using ensembles of models.
Finally, we will argue that the conclusion of TC’s article — namely that we want the original and are disappointed with a lossy compression version — is a straw man argument, as it oversimplifies the capabilities and goals of language models. The goal of language models per se is not to preserve all details, but to produce coherent and relevant text based on a prompt.
Let us begin with some general remarks on the rhetorical aspects of the article.
A misleading framework
First, the author frames the debate, and imposes an analogy which constrains the capabilities of language models by comparing them to a lossy compression algorithm. This framing overlooks the fact that language models have many other capabilities, and it gets worse when the author then launches his attacks on such “compressive LLMs”. In rhetoric, we call that the straw man fallacy.
Imagine someone telling you that the laws of physics evidently do not contain projectiles and flowers, which therefore means the laws of physics are lossy compressions of the world, and because they are lossy yet plausible, then they both miss something and are misleading, therefore they’re just a distraction. Right…
Implicit assumptions
The article implicitly assumes that we want to preserve all details in the information retrieved from the result of a process, without considering that the goal of a process may be to simplify or abstract information.
It also works against its own goal of pointing out unsolvable problems in language models by showing how a problem in lossy compression was solved by Xerox, highlighting that the process of correction and improvement is ongoing and that solutions can be found for problems that arise. The article also implies that there will always be problems and that the correction process is endless, ignoring the possibility of convergence. Additionally, the article ignores the fact that countermeasures can be taken to address quirks in the process.
Once we are completely taken aboard that weak analogy with lossy compression, we are then led to the conclusion that: “we want the original, yet we are given a lossy compressed version, so we are disappointed.” Not really convincing.
All in all, the article is very well written, with a story “once upon a time, a company needed to Xerox…”, but it is no more than a fiction, a science fiction.
Let us now take a look at the technical aspects.
Blind spots are not unavoidable
Again, language models such as GPT-3, are designed to compress a whole corpus of text into a form from which sense can be made. This means that the language model is trained on a large amount of text data and uses this information to generate contextually appropriate and coherent responses to user inputs.
Of course, language models may have limitations or blind spots, but these are not inherent to the model itself. Instead, these are a result of the training data and the model architecture used. For example, if the training data contains biases or lacks certain perspectives, these biases may be reflected in the responses generated by the language model. Similarly, the model architecture used can influence the capabilities and limitations of the language model. Furthermore, no one claims that LLMs all by themselves will necessarily suffice for AGI, and there is no reason why LLMs could not be merged with other approaches, in a similar way to the modules hypothesis of human cognition as put forward by Noam Chomsky.
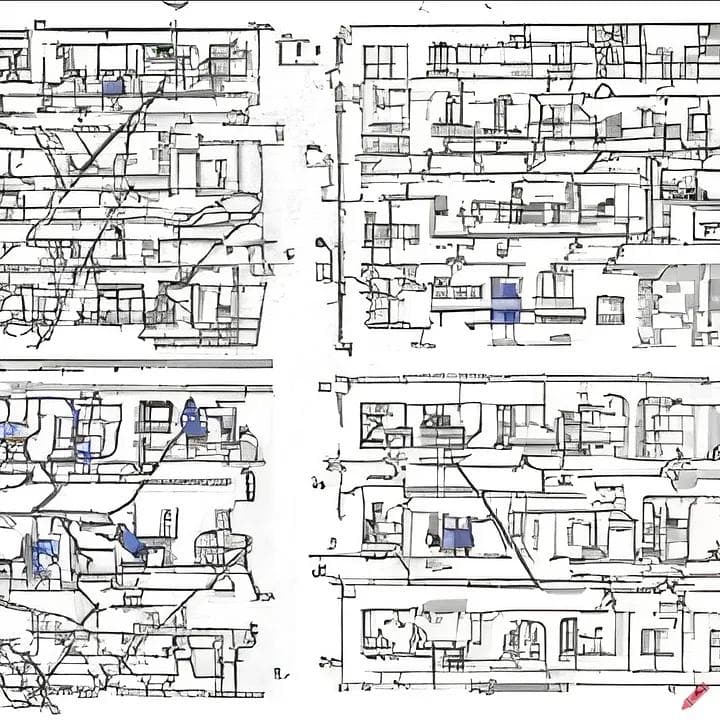
Different metrics
Language models and lossy compression algorithms differ in how they approach sequences of words. Language models, such as GPT-3, use probabilities of sequences of words to generate responses. This means that the model is trained to predict the likelihood of a sequence of words given a prompt, allowing it to generate contextually appropriate and coherent responses.
In contrast, lossy compression algorithms do not use probabilities of sequences of words. Instead, they use similarity between words to reduce the size of digital data, such as images. This means that lossy compression algorithms identify similar patterns in the data and remove redundant or less noticeable information, without considering the sequence of words.
Usefulness of summaries
Quite apart from the validity of comparing chatGPT et al. to lossy compression, the article’s last sentence asks if lossy compression is useful when you have the original data: this is similar to asking if a summary of a book is useful when you have the original! Of course, just like summaries of books can be useful even if you have the original, lossy compression algorithms can also be useful in certain situations.
Lossy compression algorithms can be useful for reducing the size of digital data, such as images, making it easier to store and transfer. This can be particularly important in situations where storage or bandwidth is limited. Additionally, lossy compression algorithms can also improve processing speed by reducing the amount of data that needs to be processed.
- test1
- test2
- test3
- test4
- test7